Connecting Gaze, Scene, and Attention Generalized Attention Estimation via Joint Modeling of Gaze and Scene Saliency (ECCV2018)

Introduction
- 透過深度學習的方式判斷人專注在圖像座標上的哪些地方,因為之前的資料集只有標註人頭和人看的位置,如果不是看圖像內的物件則沒有標註,因此作者將 MMDB 和 GazeFollow datasets 進行了額外的標註,把往外看物件的標示為 outside,才能處理如下圖 (b)(c) 的問題,將其 likelihood map 趨近於 0,而如果目標在圖像內則輸出其座標和 likelihood map,此外兩者都會輸出其 3D gaze angle。
our work predicts a fixation likelihood map of the scene as well as whether the person is looking at a location inside or outside of the image.

Method

Inputs:
- The whole image
- A crop of the subject’s face
- The location of her face
Outputs:
- yaw and pitch degrees
- heatmap
- How likely
架構細節
- 這些各種顏色的 conv 主要是用來降低 feature 維度,face 輸出的 16 維是歐拉角的旋轉矩陣,而 heatmap 的 ground 把長寬座標各分為 10 個 grid 的 xy 座標。

- 這篇的 Loss 都沒給公式,角度的部分如下圖三是計算投影到影像空間中的 cosine distance 當 L1 loss,作者稱其為 project and compare loss,另外兩個部分則是使用 cross entropy。

- 因為資料集有些有角度有些沒有,有些只有 outside 的資料,因此訓練時只對應有資料集的部分網路做訓練,其他地方就會先 freeze 住。

Experiment
Person-Dependent Saliency Prediction
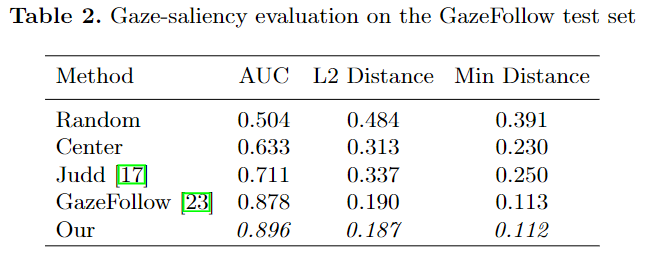
Gaze Angle Prediction

Generalized Attention Prediction During Naturalistic Social
Interactions

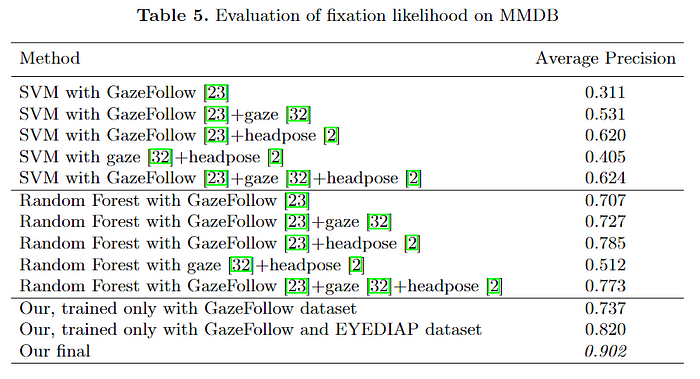
Alternative Model and Diagnostics
